The prestigious 2024 Nobel Prize in Chemistry has been awarded to three pioneers in the field of computational structural biology: David Baker, Demis Hassabis, and John Jumper. This recognition underscores the growing importance of artificial intelligence in life sciences.
While Jumper and Hassabis are responsible for the AlphaFold project for the prediction of protein structures starting from their sequence of amino acids, David Backer was awarded for advancing the field of protein design.
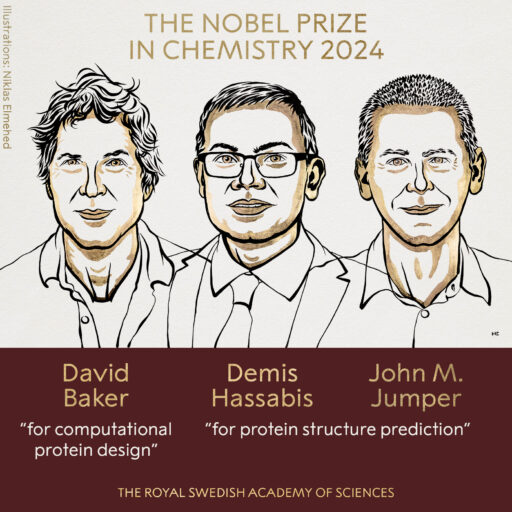
Structure prediction implies the determination of the three-dimensional coordinates of the atoms of a protein starting from the one-dimensional information of its sequence of amino acids (group of atoms). By definition, the problem is ill-posed, i.e. there are a number of hidden parameters that are required to identify a function (a model) that can transform 1D data into 3D. The best software to solve the problem has been implemented by the DeepMind laboratory, headed by Demis Hssabis, and it is called AlphaFold (AF).
Protein design is another aspect of structural biology and enables scientists to design synthetic proteins with a set of desired properties, including the design of binding sites for specific substrates, design of enzymes with custom functions and many other applications, including structure-based drug discovery. Best methods to effective designs are available thanks to the contributions of David Backer to the field.
In the future, we will be able to directly model interactions between proteins in the context of the cell, and predict the phenotypic effects of changes in the proteins and the media. This would enable us to understand many diseases whose mechanisms are still unclear — such as Alzheimer’s or Parkinson’s disease, some of the most common proteinopathies.
The astonishing results obtained by AF have been possible thanks to the Critical Assessment of Protein Structure (CASP) initiative that helped in defining the problem and developing a critical evaluation to measure the advancement of the field in the most unbiased possible way.
AI and deep learning models are impractical without quality training data. The experimental community has played a crucial role over the past 50 years by dedicating significant effort to providing high-quality structures for numerous intriguing proteins. Additionally, the availability of sequence data for billions of proteins has greatly contributed to this progress.
Since AlphaFold (AF) has been integrated into EBI services (AlphaFoldDB, UniProtKB, PDBe, …), AI-predicted protein structures of all known protein sequences are available to the scientific community. AF predictions revealed that a large fraction of protein regions are not predicted with high confidence and are represented as spaghetti structures wrapping around globular domains. These regions are intrinsically disordered, i.e. they function without adopting a well-defined three-dimensional structure.
The contribution of the BioComputingUP laboratory at the Dept. of Biomedical Sciences was to provide a critical evaluation of the ability of computational models to predict disordered regions by organising a challenge, the Critical Assessment of Intrinsic protein Disorder (CAID) that is similar to CASP. Our work has been recognized and used by AlphaFold to assess its limitations as reported in the corresponding publications. Moreover, we devised a way to exploit AF predictions to identify conditionally folding regions which are very likely to be functional and prone to bind other molecules.