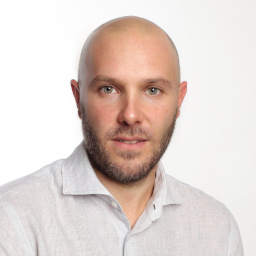
ACADEMIC PROFILES
SOCIAL
REPOSITORIES
CONTACTS
+39 049 827 6260
+39 049 827 6269
Journal Articles
2025
Aspromonte M C; Conte A D; Zhu S; Tan W; Shen Y; Zhang Y; Li Q; Wang M H; Babbi G; Bovo S; Martelli P L; Casadio R; Althagafi A; Toonsi S; Kulmanov M; Hoehndorf R; Katsonis P; Williams A; Lichtarge O; Xian S; Surento W; Pejaver V; Mooney S D; Sunderam U; Srinivasan R; Murgia A; Piovesan D; Tosatto S C E; Leonardi E
CAGI6 ID panel challenge: assessment of phenotype and variant predictions in 415 children with neurodevelopmental disorders (NDDs) Journal Article
In: Human Genetics, 2025, (Cited by: 1; Open Access).
Abstract | Altmetric | Dimensions | PlumX | Links:
@article{SCOPUS_ID:85217180047,
title = {CAGI6 ID panel challenge: assessment of phenotype and variant predictions in 415 children with neurodevelopmental disorders (NDDs)},
author = {Maria Cristina Aspromonte and Alessio Del Conte and Shaowen Zhu and Wuwei Tan and Yang Shen and Yexian Zhang and Qi Li and Maggie Haitian Wang and Giulia Babbi and Samuele Bovo and Pier Luigi Martelli and Rita Casadio and Azza Althagafi and Sumyyah Toonsi and Maxat Kulmanov and Robert Hoehndorf and Panagiotis Katsonis and Amanda Williams and Olivier Lichtarge and Su Xian and Wesley Surento and Vikas Pejaver and Sean D. Mooney and Uma Sunderam and Rajgopal Srinivasan and Alessandra Murgia and Damiano Piovesan and Silvio C. E. Tosatto and Emanuela Leonardi},
url = {https://www.scopus.com/record/display.uri?eid=2-s2.0-85217180047&origin=inward},
doi = {10.1007/s00439-024-02722-w},
year = {2025},
date = {2025-01-01},
journal = {Human Genetics},
publisher = {Springer Science and Business Media Deutschland GmbH},
abstract = {© The Author(s) 2025.The Genetics of Neurodevelopmental Disorders Lab in Padua provided a new intellectual disability (ID) Panel challenge for computational methods to predict patient phenotypes and their causal variants in the context of the Critical Assessment of the Genome Interpretation, 6th edition (CAGI6). Eight research teams submitted a total of 30 models to predict phenotypes based on the sequences of 74 genes (VCF format) in 415 pediatric patients affected by Neurodevelopmental Disorders (NDDs). NDDs are clinically and genetically heterogeneous conditions, with onset in infant age. Here, we assess the ability and accuracy of computational methods to predict comorbid phenotypes based on clinical features described in each patient and their causal variants. We also evaluated predictions for possible genetic causes in patients without a clear genetic diagnosis. Like the previous ID Panel challenge in CAGI5, seven clinical features (ID, ASD, ataxia, epilepsy, microcephaly, macrocephaly, hypotonia), and variants (Pathogenic/Likely Pathogenic, Variants of Uncertain Significance and Risk Factors) were provided. The phenotypic traits and variant data of 150 patients from the CAGI5 ID Panel Challenge were provided as training set for predictors. The CAGI6 challenge confirms CAGI5 results that predicting phenotypes from gene panel data is highly challenging, with AUC values close to random, and no method able to predict relevant variants with both high accuracy and precision. However, a significant improvement is noted for the best method, with recall increasing from 66% to 82%. Several groups also successfully predicted difficult-to-detect variants, emphasizing the importance of variants initially excluded by the Padua NDD Lab.},
note = {Cited by: 1; Open Access},
keywords = {},
pubstate = {published},
tppubtype = {article}
}
Han K; Song S; Pak M; Kim C; Ri C; Conte A D; Piovesan D
PredIDR: Accurate prediction of protein intrinsic disorder regions using deep convolutional neural network Journal Article
In: International Journal of Biological Macromolecules, vol. 284, 2025, (Cited by: 0; Open Access).
Abstract | Altmetric | Dimensions | PlumX | Links:
@article{SCOPUS_ID:85210122158,
title = {PredIDR: Accurate prediction of protein intrinsic disorder regions using deep convolutional neural network},
author = {Kun-Sop Han and Se-Ryong Song and Myong-hyon Pak and Chol-Song Kim and Chol-Pyok Ri and Alessio Del Conte and Damiano Piovesan},
url = {https://www.scopus.com/record/display.uri?eid=2-s2.0-85210122158&origin=inward},
doi = {10.1016/j.ijbiomac.2024.137665},
year = {2025},
date = {2025-01-01},
journal = {International Journal of Biological Macromolecules},
volume = {284},
publisher = {Elsevier B.V.},
abstract = {© 2024 Elsevier B.V.The involvement of protein intrinsic disorder in essential biological processes, it is well known in structural biology. However, experimental methods for detecting intrinsic structural disorder and directly measuring highly dynamic behavior of protein structure are limited. To address this issue, several computational methods to predict intrinsic disorder from protein sequences were developed and their performance is evaluated by the Critical Assessment of protein Intrinsic Disorder (CAID). In this paper, we describe a new computational method, PredIDR, which provides accurate prediction of intrinsically disordered regions in proteins, mimicking experimental X-ray missing residues. Indeed, missing residues in Protein Data Bank (PDB) were used as positive examples to train a deep convolutional neural network which produces two types of output for short and long regions. PredIDR took part in the second round of CAID and was as accurate as the top state-of-the-art IDR prediction methods. PredIDR can be freely used through the CAID Prediction Portal available at https://caid.idpcentral.org/portal or downloaded as a Singularity container from https://biocomputingup.it/shared/caid-predictors/.},
note = {Cited by: 0; Open Access},
keywords = {},
pubstate = {published},
tppubtype = {article}
}
Blum M; Andreeva A; Florentino L C; Chuguransky S R; Grego T; Hobbs E; Pinto B L; Orr A; Paysan-Lafosse T; Ponamareva I; Salazar G A; Bordin N; Bork P; Bridge A; Colwell L; Gough J; Haft D H; Letunic I; Llinares-López F; Marchler-Bauer A; Meng-Papaxanthos L; Mi H; Natale D A; Orengo C A; Pandurangan A P; Piovesan D; Rivoire C; Sigrist C J A; Thanki N; Thibaud-Nissen F; Thomas P D; Tosatto S C E; Wu C H; Bateman A
InterPro: The protein sequence classification resource in 2025 Journal Article
In: Nucleic Acids Research, vol. 53, no. D1, pp. D444-D456, 2025, (Cited by: 4; Open Access).
Abstract | Altmetric | Dimensions | PlumX | Links:
@article{SCOPUS_ID:85214359849,
title = {InterPro: The protein sequence classification resource in 2025},
author = {Matthias Blum and Antonina Andreeva and Laise Cavalcanti Florentino and Sara Rocio Chuguransky and Tiago Grego and Emma Hobbs and Beatriz Lazaro Pinto and Ailsa Orr and Typhaine Paysan-Lafosse and Irina Ponamareva and Gustavo A Salazar and Nicola Bordin and Peer Bork and Alan Bridge and Lucy Colwell and Julian Gough and Daniel H Haft and Ivica Letunic and Felipe Llinares-López and Aron Marchler-Bauer and Laetitia Meng-Papaxanthos and Huaiyu Mi and Darren A Natale and Christine A Orengo and Arun P Pandurangan and Damiano Piovesan and Catherine Rivoire and Christian J. A Sigrist and Narmada Thanki and Françoise Thibaud-Nissen and Paul D Thomas and Silvio C. E Tosatto and Cathy H Wu and Alex Bateman},
url = {https://www.scopus.com/record/display.uri?eid=2-s2.0-85214359849&origin=inward},
doi = {10.1093/nar/gkae1082},
year = {2025},
date = {2025-01-01},
journal = {Nucleic Acids Research},
volume = {53},
number = {D1},
pages = {D444-D456},
publisher = {Oxford University Press},
abstract = {© 2025 The Author(s) 2024.InterPro (https://www.ebi.ac.uk/interpro) is a freely accessible resource for the classification of protein sequences into families. It integrates predictive models, known as signatures, from multiple member databases to classify sequences into families and predict the presence of domains and significant sites. The InterPro database provides annotations for over 200 million sequences, ensuring extensive coverage of UniProtKB, the standard repository of protein sequences, and includes mappings to several other major resources, such as Gene Ontology (GO), Protein Data Bank in Europe (PDBe) and the AlphaFold Protein Structure Database. In this publication, we report on the status of InterPro (version 101.0), detailing new developments in the database, associated web interface and software. Notable updates include the increased integration of structures predicted by AlphaFold and the enhanced description of protein families using artificial intelligence. Over the past two years, more than 5000 new InterPro entries have been created. The InterPro website now offers access to 85 000 protein families and domains from its member databases and serves as a long-Term archive for retired databases. InterPro data, software and tools are freely available.},
note = {Cited by: 4; Open Access},
keywords = {},
pubstate = {published},
tppubtype = {article}
}
Piovesan D; Conte A D; Mehdiabadi M; Aspromonte M C; Blum M; Tesei G; Bülow S; Lindorff-Larsen K; Tosatto S C E
MOBIDB in 2025: integrating ensemble properties and function annotations for intrinsically disordered proteins Journal Article
In: Nucleic Acids Research, vol. 53, no. D1, pp. D495-D503, 2025, (Cited by: 2; Open Access).
Abstract | Altmetric | Dimensions | PlumX | Links:
@article{SCOPUS_ID:85213063415,
title = {MOBIDB in 2025: integrating ensemble properties and function annotations for intrinsically disordered proteins},
author = {Damiano Piovesan and Alessio Del Conte and Mahta Mehdiabadi and Maria Cristina Aspromonte and Matthias Blum and Giulio Tesei and Sören Bülow and Kresten Lindorff-Larsen and Silvio C. E. Tosatto},
url = {https://www.scopus.com/record/display.uri?eid=2-s2.0-85213063415&origin=inward},
doi = {10.1093/nar/gkae969},
year = {2025},
date = {2025-01-01},
journal = {Nucleic Acids Research},
volume = {53},
number = {D1},
pages = {D495-D503},
publisher = {Oxford University Press},
abstract = {© The Author(s) 2024. Published by Oxford University Press on behalf of Nucleic Acids Research.The MobiDB database (URL: https://mobidb.org/) aims to provide structural and functional information about intrinsic protein disorder, aggregating annotations from the literature, experimental data, and predictions for all known protein sequences. Here, we describe the improvements made to our resource to capture more information, simplify access to the aggregated data, and increase documentation of all MobiDB features. Compared to the previous release, all underlying pipeline modules were updated. The prediction module is ten times faster and can detect if a predicted disordered region is structurally extended or compact. The PDB component is now able to process large cryo-EM structures extending the number of processed entries. The entry page has been restyled to highlight functional aspects of disorder and all graphical modules have been completely reimplemented for better flexibility and faster rendering. The server has been improved to optimise bulk downloads. Annotation provenance has been standardised by adopting ECO terms. Finally, we propagated disorder function (IDPO and GO terms) from the DisProt database exploiting sequence similarity and protein embeddings. These improvements, along with the addition of comprehensive training material, offer a more intuitive interface and novel functional knowledge about intrinsic disorder.},
note = {Cited by: 2; Open Access},
keywords = {},
pubstate = {published},
tppubtype = {article}
}
Aspromonte M C; Conte A D; Polli R; Baldo D; Benedicenti F; Bettella E; Bigoni S; Boni S; Ciaccio C; D’Arrigo S; Donati I; Granocchio E; Mammi I; Milani D; Negrin S; Nosadini M; Soli F; Stanzial F; Turolla L; Piovesan D; Tosatto S C E; Murgia A; Leonardi E
Genetic variants and phenotypic data curated for the CAGI6 intellectual disability panel challenge Journal Article
In: Human Genetics, 2025, (Cited by: 0; Open Access).
Abstract | Altmetric | Dimensions | PlumX | Links:
@article{SCOPUS_ID:86000084600,
title = {Genetic variants and phenotypic data curated for the CAGI6 intellectual disability panel challenge},
author = {Maria Cristina Aspromonte and Alessio Del Conte and Roberta Polli and Demetrio Baldo and Francesco Benedicenti and Elisa Bettella and Stefania Bigoni and Stefania Boni and Claudia Ciaccio and Stefano D’Arrigo and Ilaria Donati and Elisa Granocchio and Isabella Mammi and Donatella Milani and Susanna Negrin and Margherita Nosadini and Fiorenza Soli and Franco Stanzial and Licia Turolla and Damiano Piovesan and Silvio C. E. Tosatto and Alessandra Murgia and Emanuela Leonardi},
url = {https://www.scopus.com/record/display.uri?eid=2-s2.0-86000084600&origin=inward},
doi = {10.1007/s00439-025-02733-1},
year = {2025},
date = {2025-01-01},
journal = {Human Genetics},
publisher = {Springer Science and Business Media Deutschland GmbH},
abstract = {© The Author(s) 2025.Neurodevelopmental disorders (NDDs) are common conditions including clinically diverse and genetically heterogeneous diseases, such as intellectual disability, autism spectrum disorders, and epilepsy. The intricate genetic underpinnings of NDDs pose a formidable challenge, given their multifaceted genetic architecture and heterogeneous clinical presentations. This work delves into the intricate interplay between genetic variants and phenotypic manifestations in neurodevelopmental disorders, presenting a dataset curated for the Critical Assessment of Genome Interpretation (CAGI6) ID Panel Challenge. The CAGI6 competition serves as a platform for evaluating the efficacy of computational methods in predicting phenotypic outcomes from genetic data. In this study, a targeted gene panel sequencing has been used to investigate the genetic causes of NDDs in a cohort of 415 paediatric patients. We identified 60 pathogenic and 49 likely pathogenic variants in 102 individuals that accounted for 25% of NDD cases in the cohort. The most mutated genes were ANKRD11, MECP2, ARID1B, ASH1L, CHD8, KDM5C, MED12 and PTCHD1 The majority of pathogenic variants were de novo, with some inherited from mildly affected parents. Loss-of-function variants were the most common type of pathogenic variant. In silico analysis tools were used to assess the potential impact of variants on splicing and structural/functional effects of missense variants. The study highlights the challenges in variant interpretation especially in cases with atypical phenotypic manifestations. Overall, this study provides valuable insights into the genetic causes of NDDs and emphasises the importance of understanding the underlying genetic factors for accurate diagnosis, and intervention development in neurodevelopmental conditions.},
note = {Cited by: 0; Open Access},
keywords = {},
pubstate = {published},
tppubtype = {article}
}