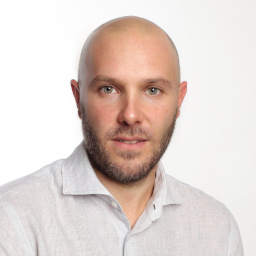
ACADEMIC PROFILES
SOCIAL
REPOSITORIES
CONTACTS
+39 049 827 6260
+39 049 827 6269
BIOGRAPHY
Damiano Piovesan is currently Associate Professor in Bioinformatics (SSD BIO/10)at the Department of Biomedical Sciences of the University of Padua (Italy). He specializes in protein function prediction with expertise on bioinformatics tools and methods.
ACADEMIC POSITION
Associate professor
(since 03/2022)
ACADEMIC CAREER & DEGREES
- 2022 – Assistant Professor – Department of Biomedical Sciences
University of Padua – Italy
- 2019 – PostDoc researcher – Department of Biomedical Sciences
University of Padua – Italy - 2013 – PhD in Biotechnology, pharmacology and toxicology
University of Bologna – Italy - 2009 – MSc in Bioinformatics
University of Bologna – Italy - 2007 – BSc in Biotechnology
University of Bologna – Italy
LANGUAGES
English
Italian
(Upper Intermediate)
(Native)
2025
Journal Articles
Damiano Piovesan; Alessio Del Conte; Mahta Mehdiabadi; Maria Cristina Aspromonte; Matthias Blum; Giulio Tesei; Sören Bülow; Kresten Lindorff-Larsen; Silvio C. E. Tosatto
MOBIDB in 2025: integrating ensemble properties and function annotations for intrinsically disordered proteins Journal Article
In: Nucleic Acids Research, vol. 53, no. D1, pp. D495-D503, 2025, (Cited by: 4; Open Access).
@article{SCOPUS_ID:85213063415,
title = {MOBIDB in 2025: integrating ensemble properties and function annotations for intrinsically disordered proteins},
author = {Damiano Piovesan and Alessio Del Conte and Mahta Mehdiabadi and Maria Cristina Aspromonte and Matthias Blum and Giulio Tesei and Sören Bülow and Kresten Lindorff-Larsen and Silvio C. E. Tosatto},
url = {https://www.scopus.com/record/display.uri?eid=2-s2.0-85213063415&origin=inward},
doi = {10.1093/nar/gkae969},
year = {2025},
date = {2025-01-01},
journal = {Nucleic Acids Research},
volume = {53},
number = {D1},
pages = {D495-D503},
publisher = {Oxford University Press},
abstract = {© The Author(s) 2024. Published by Oxford University Press on behalf of Nucleic Acids Research.The MobiDB database (URL: https://mobidb.org/) aims to provide structural and functional information about intrinsic protein disorder, aggregating annotations from the literature, experimental data, and predictions for all known protein sequences. Here, we describe the improvements made to our resource to capture more information, simplify access to the aggregated data, and increase documentation of all MobiDB features. Compared to the previous release, all underlying pipeline modules were updated. The prediction module is ten times faster and can detect if a predicted disordered region is structurally extended or compact. The PDB component is now able to process large cryo-EM structures extending the number of processed entries. The entry page has been restyled to highlight functional aspects of disorder and all graphical modules have been completely reimplemented for better flexibility and faster rendering. The server has been improved to optimise bulk downloads. Annotation provenance has been standardised by adopting ECO terms. Finally, we propagated disorder function (IDPO and GO terms) from the DisProt database exploiting sequence similarity and protein embeddings. These improvements, along with the addition of comprehensive training material, offer a more intuitive interface and novel functional knowledge about intrinsic disorder.},
note = {Cited by: 4; Open Access},
keywords = {},
pubstate = {published},
tppubtype = {article}
}
Matthias Blum; Antonina Andreeva; Laise Cavalcanti Florentino; Sara Rocio Chuguransky; Tiago Grego; Emma Hobbs; Beatriz Lazaro Pinto; Ailsa Orr; Typhaine Paysan-Lafosse; Irina Ponamareva; Gustavo A Salazar; Nicola Bordin; Peer Bork; Alan Bridge; Lucy Colwell; Julian Gough; Daniel H Haft; Ivica Letunic; Felipe Llinares-López; Aron Marchler-Bauer; Laetitia Meng-Papaxanthos; Huaiyu Mi; Darren A Natale; Christine A Orengo; Arun P Pandurangan; Damiano Piovesan; Catherine Rivoire; Christian J. A Sigrist; Narmada Thanki; Françoise Thibaud-Nissen; Paul D Thomas; Silvio C. E Tosatto; Cathy H Wu; Alex Bateman
InterPro: The protein sequence classification resource in 2025 Journal Article
In: Nucleic Acids Research, vol. 53, no. D1, pp. D444-D456, 2025, (Cited by: 57; Open Access).
@article{SCOPUS_ID:85214359849,
title = {InterPro: The protein sequence classification resource in 2025},
author = {Matthias Blum and Antonina Andreeva and Laise Cavalcanti Florentino and Sara Rocio Chuguransky and Tiago Grego and Emma Hobbs and Beatriz Lazaro Pinto and Ailsa Orr and Typhaine Paysan-Lafosse and Irina Ponamareva and Gustavo A Salazar and Nicola Bordin and Peer Bork and Alan Bridge and Lucy Colwell and Julian Gough and Daniel H Haft and Ivica Letunic and Felipe Llinares-López and Aron Marchler-Bauer and Laetitia Meng-Papaxanthos and Huaiyu Mi and Darren A Natale and Christine A Orengo and Arun P Pandurangan and Damiano Piovesan and Catherine Rivoire and Christian J. A Sigrist and Narmada Thanki and Françoise Thibaud-Nissen and Paul D Thomas and Silvio C. E Tosatto and Cathy H Wu and Alex Bateman},
url = {https://www.scopus.com/record/display.uri?eid=2-s2.0-85214359849&origin=inward},
doi = {10.1093/nar/gkae1082},
year = {2025},
date = {2025-01-01},
journal = {Nucleic Acids Research},
volume = {53},
number = {D1},
pages = {D444-D456},
publisher = {Oxford University Press},
abstract = {© 2025 The Author(s) 2024.InterPro (https://www.ebi.ac.uk/interpro) is a freely accessible resource for the classification of protein sequences into families. It integrates predictive models, known as signatures, from multiple member databases to classify sequences into families and predict the presence of domains and significant sites. The InterPro database provides annotations for over 200 million sequences, ensuring extensive coverage of UniProtKB, the standard repository of protein sequences, and includes mappings to several other major resources, such as Gene Ontology (GO), Protein Data Bank in Europe (PDBe) and the AlphaFold Protein Structure Database. In this publication, we report on the status of InterPro (version 101.0), detailing new developments in the database, associated web interface and software. Notable updates include the increased integration of structures predicted by AlphaFold and the enhanced description of protein families using artificial intelligence. Over the past two years, more than 5000 new InterPro entries have been created. The InterPro website now offers access to 85 000 protein families and domains from its member databases and serves as a long-Term archive for retired databases. InterPro data, software and tools are freely available.},
note = {Cited by: 57; Open Access},
keywords = {},
pubstate = {published},
tppubtype = {article}
}
Mahta Mehdiabadi; Matthias Blum; Giulio Tesei; Soren Bulow; Kresten Lindorff-Larsen; Silvio C. E. Tosatto; Damiano Piovesan
MobiDB-lite 4.0: faster prediction of intrinsic protein disorder and structural compactness Journal Article
In: Bioinformatics, vol. 41, no. 5, 2025, (Cited by: 0; Open Access).
@article{SCOPUS_ID:105007010286,
title = {MobiDB-lite 4.0: faster prediction of intrinsic protein disorder and structural compactness},
author = {Mahta Mehdiabadi and Matthias Blum and Giulio Tesei and Soren Bulow and Kresten Lindorff-Larsen and Silvio C. E. Tosatto and Damiano Piovesan},
url = {https://www.scopus.com/record/display.uri?eid=2-s2.0-105007010286&origin=inward},
doi = {10.1093/bioinformatics/btaf297},
year = {2025},
date = {2025-01-01},
journal = {Bioinformatics},
volume = {41},
number = {5},
publisher = {Oxford University Press},
abstract = {© The Author(s) 2025. Published by Oxford University Press.Motivation: In recent years, many disorder predictors have been developed to identify intrinsically disordered regions (IDRs) in proteins, achieving high accuracy. However, it may be difficult to interpret differences in predictions across methods. Consensus methods offer a simple solution, highlighting reliable predictions while filtering out uncertain positions. Here, we present a new version of MobiDB-lite, a consensus method designed to predict long IDRs and classify them based on compositional biases and conformational properties. Results: MobiDB-lite 4.0 pipeline was optimized to be ten times faster than the previous version. It now provides compactness annotations based on predicted apparent scaling exponent. The newly added features and disorder subclassifications allow the users to get a comprehensive insight into the protein’s function and characteristics. MobiDB-lite 4.0 is integrated into the MobiDB and DisProt databases. A version without the compactness predictor is integrated into InterProScan, propagating MobiDB-lite annotations to UniProtKB. Availability and implementation: The MobiDB-lite 4.0 source code and a Docker container are available from the GitHub repository: https://github.com/BioComputingUP/MobiDB-lite.},
note = {Cited by: 0; Open Access},
keywords = {},
pubstate = {published},
tppubtype = {article}
}
Kun-Sop Han; Se-Ryong Song; Myong-hyon Pak; Chol-Song Kim; Chol-Pyok Ri; Alessio Del Conte; Damiano Piovesan
PredIDR: Accurate prediction of protein intrinsic disorder regions using deep convolutional neural network Journal Article
In: International Journal of Biological Macromolecules, vol. 284, 2025, (Cited by: 1; Open Access).
@article{SCOPUS_ID:85210122158,
title = {PredIDR: Accurate prediction of protein intrinsic disorder regions using deep convolutional neural network},
author = {Kun-Sop Han and Se-Ryong Song and Myong-hyon Pak and Chol-Song Kim and Chol-Pyok Ri and Alessio Del Conte and Damiano Piovesan},
url = {https://www.scopus.com/record/display.uri?eid=2-s2.0-85210122158&origin=inward},
doi = {10.1016/j.ijbiomac.2024.137665},
year = {2025},
date = {2025-01-01},
journal = {International Journal of Biological Macromolecules},
volume = {284},
publisher = {Elsevier B.V.},
abstract = {© 2024 Elsevier B.V.The involvement of protein intrinsic disorder in essential biological processes, it is well known in structural biology. However, experimental methods for detecting intrinsic structural disorder and directly measuring highly dynamic behavior of protein structure are limited. To address this issue, several computational methods to predict intrinsic disorder from protein sequences were developed and their performance is evaluated by the Critical Assessment of protein Intrinsic Disorder (CAID). In this paper, we describe a new computational method, PredIDR, which provides accurate prediction of intrinsically disordered regions in proteins, mimicking experimental X-ray missing residues. Indeed, missing residues in Protein Data Bank (PDB) were used as positive examples to train a deep convolutional neural network which produces two types of output for short and long regions. PredIDR took part in the second round of CAID and was as accurate as the top state-of-the-art IDR prediction methods. PredIDR can be freely used through the CAID Prediction Portal available at https://caid.idpcentral.org/portal or downloaded as a Singularity container from https://biocomputingup.it/shared/caid-predictors/.},
note = {Cited by: 1; Open Access},
keywords = {},
pubstate = {published},
tppubtype = {article}
}
Maria Cristina Aspromonte; Alessio Del Conte; Shaowen Zhu; Wuwei Tan; Yang Shen; Yexian Zhang; Qi Li; Maggie Haitian Wang; Giulia Babbi; Samuele Bovo; Pier Luigi Martelli; Rita Casadio; Azza Althagafi; Sumyyah Toonsi; Maxat Kulmanov; Robert Hoehndorf; Panagiotis Katsonis; Amanda Williams; Olivier Lichtarge; Su Xian; Wesley Surento; Vikas Pejaver; Sean D. Mooney; Uma Sunderam; Rajgopal Srinivasan; Alessandra Murgia; Damiano Piovesan; Silvio C. E. Tosatto; Emanuela Leonardi
CAGI6 ID panel challenge: assessment of phenotype and variant predictions in 415 children with neurodevelopmental disorders (NDDs) Journal Article
In: Human Genetics, vol. 144, no. 2, pp. 227-242, 2025, (Cited by: 1; Open Access).
@article{SCOPUS_ID:85217180047,
title = {CAGI6 ID panel challenge: assessment of phenotype and variant predictions in 415 children with neurodevelopmental disorders (NDDs)},
author = {Maria Cristina Aspromonte and Alessio Del Conte and Shaowen Zhu and Wuwei Tan and Yang Shen and Yexian Zhang and Qi Li and Maggie Haitian Wang and Giulia Babbi and Samuele Bovo and Pier Luigi Martelli and Rita Casadio and Azza Althagafi and Sumyyah Toonsi and Maxat Kulmanov and Robert Hoehndorf and Panagiotis Katsonis and Amanda Williams and Olivier Lichtarge and Su Xian and Wesley Surento and Vikas Pejaver and Sean D. Mooney and Uma Sunderam and Rajgopal Srinivasan and Alessandra Murgia and Damiano Piovesan and Silvio C. E. Tosatto and Emanuela Leonardi},
url = {https://www.scopus.com/record/display.uri?eid=2-s2.0-85217180047&origin=inward},
doi = {10.1007/s00439-024-02722-w},
year = {2025},
date = {2025-01-01},
journal = {Human Genetics},
volume = {144},
number = {2},
pages = {227-242},
publisher = {Springer Science and Business Media Deutschland GmbH},
abstract = {© The Author(s) 2025.The Genetics of Neurodevelopmental Disorders Lab in Padua provided a new intellectual disability (ID) Panel challenge for computational methods to predict patient phenotypes and their causal variants in the context of the Critical Assessment of the Genome Interpretation, 6th edition (CAGI6). Eight research teams submitted a total of 30 models to predict phenotypes based on the sequences of 74 genes (VCF format) in 415 pediatric patients affected by Neurodevelopmental Disorders (NDDs). NDDs are clinically and genetically heterogeneous conditions, with onset in infant age. Here, we assess the ability and accuracy of computational methods to predict comorbid phenotypes based on clinical features described in each patient and their causal variants. We also evaluated predictions for possible genetic causes in patients without a clear genetic diagnosis. Like the previous ID Panel challenge in CAGI5, seven clinical features (ID, ASD, ataxia, epilepsy, microcephaly, macrocephaly, hypotonia), and variants (Pathogenic/Likely Pathogenic, Variants of Uncertain Significance and Risk Factors) were provided. The phenotypic traits and variant data of 150 patients from the CAGI5 ID Panel Challenge were provided as training set for predictors. The CAGI6 challenge confirms CAGI5 results that predicting phenotypes from gene panel data is highly challenging, with AUC values close to random, and no method able to predict relevant variants with both high accuracy and precision. However, a significant improvement is noted for the best method, with recall increasing from 66% to 82%. Several groups also successfully predicted difficult-to-detect variants, emphasizing the importance of variants initially excluded by the Padua NDD Lab.},
note = {Cited by: 1; Open Access},
keywords = {},
pubstate = {published},
tppubtype = {article}
}