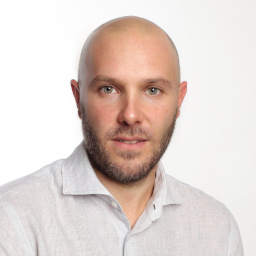
BIOGRAPHY
Damiano Piovesan is currently Associate Professor in Bioinformatics (SSD BIO/10)at the Department of Biomedical Sciences of the University of Padua (Italy). He specializes in protein function prediction with expertise on bioinformatics tools and methods.
ACADEMIC POSITION
Associate professor
(since 03/2022)
DEGREES
- 2019 –
- 2010 –
LANGUAGES
English
Italian
(Upper Intermediate)
(Native)
2024
Journal Articles
Alessio Del Conte; Giorgia F Camagni; Damiano Clementel; Giovanni Minervini; Alexander Miguel Monzon; Carlo Ferrari; Damiano Piovesan; Silvio C. E Tosatto
RING 4.0: Faster residue interaction networks with novel interaction types across over 35,000 different chemical structures Journal Article
In: 2024, ISSN: 03051048, (Cited by: 5; All Open Access, Gold Open Access).
@article{DelConte2024W306,
title = {RING 4.0: Faster residue interaction networks with novel interaction types across over 35,000 different chemical structures},
author = { Alessio Del Conte and Giorgia F Camagni and Damiano Clementel and Giovanni Minervini and Alexander Miguel Monzon and Carlo Ferrari and Damiano Piovesan and Silvio C. E Tosatto},
url = {https://www.scopus.com/inward/record.uri?eid=2-s2.0-85197788039\&doi=10.1093%2fnar%2fgkae337\&partnerID=40\&md5=bca70d16fbb39f5466a3957673ef9eef},
doi = {10.1093/nar/gkae337},
issn = {03051048},
year = {2024},
date = {2024-01-01},
abstract = {Residue interaction networks (RINs) are a valuable approach for representing contacts in protein structures. RINs have been widely used in various research areas, including the analysis of mutation effects, domain-domain communication, catalytic activity, and molecular dynamics simulations. The RING server is a powerful tool to calculate non-covalent molecular interactions based on geometrical parameters, providing high-quality and reliable results. Here, we introduce RING 4.0, which includes significant enhancements for identifying both covalent and non-covalent bonds in protein structures. It now encompasses seven different interaction types, with the addition of π-hydrogen, halogen bonds and metal ion coordination sites. The definitions of all available bond types have also been refined and RING can now process the complete PDB chemical component dictionary (over 35000 different molecules) which provides atom names and covalent connectivity information for all known ligands. Optimization of the software has improved execution time by an order of magnitude. The RING web server has been redesigned to provide a more engaging and interactive user experience, incorporating new visualization tools. Users can now visualize all types of interactions simultaneously in the structure viewer and network component. The web server, including extensive help and tutorials, is available from URL: https://ring.biocomputingup.it/. © 2024 The Author(s). Published by Oxford University Press on behalf of Nucleic Acids Research.},
note = {Cited by: 5; All Open Access, Gold Open Access},
keywords = {},
pubstate = {published},
tppubtype = {article}
}
Damiano Piovesan; Davide Zago; Parnal Joshi; M. Clara De Paolis Kaluza; Mahta Mehdiabadi; Rashika Ramola; Alexander Miguel Monzon; Walter Reade; Iddo Friedberg; Predrag Radivojac; Silvio C. E. Tosatto
CAFA-evaluator: a Python tool for benchmarking ontological classification methods Journal Article
In: 2024, ISSN: 26350041, (Cited by: 2; All Open Access, Gold Open Access).
@article{Piovesan2024,
title = {CAFA-evaluator: a Python tool for benchmarking ontological classification methods},
author = { Damiano Piovesan and Davide Zago and Parnal Joshi and M. Clara De Paolis Kaluza and Mahta Mehdiabadi and Rashika Ramola and Alexander Miguel Monzon and Walter Reade and Iddo Friedberg and Predrag Radivojac and Silvio C. E. Tosatto},
url = {https://www.scopus.com/inward/record.uri?eid=2-s2.0-85188993912\&doi=10.1093%2fbioadv%2fvbae043\&partnerID=40\&md5=b6e09ea188a60708097f5bc31ba115dd},
doi = {10.1093/bioadv/vbae043},
issn = {26350041},
year = {2024},
date = {2024-01-01},
abstract = {We present CAFA-evaluator, a powerful Python program designed to evaluate the performance of prediction methods on targets with hierarchical concept dependencies. It generalizes multi-label evaluation to modern ontologies where the prediction targets are drawn from a directed acyclic graph and achieves high efficiency by leveraging matrix computation and topological sorting. The program requirements include a small number of standard Python libraries, making CAFA-evaluator easy to maintain. The code replicates the Critical Assessment of protein Function Annotation (CAFA) benchmarking, which evaluates predictions of the consistent subgraphs in Gene Ontology. Owing to its reliability and accuracy, the organizers have selected CAFA-evaluator as the official CAFA evaluation software. © 2024 The Author(s). Published by Oxford University Press.},
note = {Cited by: 2; All Open Access, Gold Open Access},
keywords = {},
pubstate = {published},
tppubtype = {article}
}
Federica Quaglia; Anastasia Chasapi; Maria Victoria Nugnes; Maria Cristina Aspromonte; Emanuela Leonardi; Damiano Piovesan; Silvio C.E. Tosatto
Best practices for the manual curation of intrinsically disordered proteins in DisProt Journal Article
In: 2024, ISSN: 17580463, (Cited by: 1; All Open Access, Gold Open Access).
@article{Quaglia2024,
title = {Best practices for the manual curation of intrinsically disordered proteins in DisProt},
author = { Federica Quaglia and Anastasia Chasapi and Maria Victoria Nugnes and Maria Cristina Aspromonte and Emanuela Leonardi and Damiano Piovesan and Silvio C.E. Tosatto},
url = {https://www.scopus.com/inward/record.uri?eid=2-s2.0-85188297172\&doi=10.1093%2fdatabase%2fbaae009\&partnerID=40\&md5=df4fca19479789139b3fd19bb35c817f},
doi = {10.1093/database/baae009},
issn = {17580463},
year = {2024},
date = {2024-01-01},
abstract = {The DisProt database is a resource containing manually curated data on experimentally validated intrinsically disordered proteins (IDPs) and intrinsically disordered regions (IDRs) from the literature. Developed in 2005, its primary goal was to collect structural and functional information into proteins that lack a fixed three-dimensional structure.Today, DisProt has evolved into a major repository that not only collects experimental data but also contributes to our understanding of the IDPs/IDRs roles in various biological processes, such as autophagy or the life cycle mechanisms in viruses or their involvement in diseases (such as cancer and neurodevelopmental disorders). DisProt offers detailed information on the structural states of IDPs/IDRs, including state transitions, interactions and their functions, all provided as curated annotations. One of the central activities of DisProt is the meticulous curation of experimental data from the literature. For this reason, to ensure that every expert and volunteer curator possesses the requisite knowledge for data evaluation, collection and integration, training courses and curation materials are available. However, biocuration guidelines concur on the importance of developing robust guidelines that not only provide critical information about data consistency but also ensure data acquisition.This guideline aims to provide both biocurators and external users with best practices for manually curating IDPs and IDRs in DisProt. It describes every step of the literature curation process and provides use cases of IDP curation within DisProt. © The Author(s) 2024. Published by Oxford University Press.},
note = {Cited by: 1; All Open Access, Gold Open Access},
keywords = {},
pubstate = {published},
tppubtype = {article}
}
Maria Cristina Aspromonte; Maria Victoria Nugnes; Federica Quaglia; Adel Bouharoua; Silvio C.E. Tosatto; Damiano Piovesan; Vasileios Sagris; Vasilis J. Promponas; Anastasia Chasapi; Erzsébet Fichó; Galo E. Balatti; Gustavo Parisi; Martín González Buitrón; Gabor Erdos; Matyas Pajkos; Zsuzsanna Dosztányi; Laszlo Dobson; Alessio Del Conte; Damiano Clementel; Edoardo Salladini; Emanuela Leonardi; Fatemeh Kordevani; Hamidreza Ghafouri; Luiggi G. Tenorio Ku; Alexander Miguel Monzon; Carlo Ferrari; Zsófia Kálmán; Juliet F. Nilsson; Jaime Santos; Carlos Pintado-Grima; Salvador Ventura; Veronika Ács; Rita Pancsa; Mariane Goncalves Kulik; Miguel A. Andrade-Navarro; Pedro José Barbosa Pereira; Sonia Longhi; Philippe Le Mercier; Julian Bergier; Peter Tompa; Tamas Lazar
DisProt in 2024: improving function annotation of intrinsically disordered proteins Journal Article
In: 2024, ISSN: 03051048, (Cited by: 10; All Open Access, Gold Open Access).
@article{Aspromonte2024D434,
title = {DisProt in 2024: improving function annotation of intrinsically disordered proteins},
author = { Maria Cristina Aspromonte and Maria Victoria Nugnes and Federica Quaglia and Adel Bouharoua and Silvio C.E. Tosatto and Damiano Piovesan and Vasileios Sagris and Vasilis J. Promponas and Anastasia Chasapi and Erzs\'{e}bet Fich\'{o} and Galo E. Balatti and Gustavo Parisi and Mart\'{i}n Gonz\'{a}lez Buitr\'{o}n and Gabor Erdos and Matyas Pajkos and Zsuzsanna Doszt\'{a}nyi and Laszlo Dobson and Alessio Del Conte and Damiano Clementel and Edoardo Salladini and Emanuela Leonardi and Fatemeh Kordevani and Hamidreza Ghafouri and Luiggi G. Tenorio Ku and Alexander Miguel Monzon and Carlo Ferrari and Zs\'{o}fia K\'{a}lm\'{a}n and Juliet F. Nilsson and Jaime Santos and Carlos Pintado-Grima and Salvador Ventura and Veronika \'{A}cs and Rita Pancsa and Mariane Goncalves Kulik and Miguel A. Andrade-Navarro and Pedro Jos\'{e} Barbosa Pereira and Sonia Longhi and Philippe Le Mercier and Julian Bergier and Peter Tompa and Tamas Lazar},
url = {https://www.scopus.com/inward/record.uri?eid=2-s2.0-85176208048\&doi=10.1093%2fnar%2fgkad928\&partnerID=40\&md5=fc34ce08667ff42029fdb54d5142c08f},
doi = {10.1093/nar/gkad928},
issn = {03051048},
year = {2024},
date = {2024-01-01},
abstract = {DisProt (URL: https://disprot.org) is the gold standard database for intrinsically disordered proteins and regions, providing valuable information about their functions. The latest version of DisProt brings significant advancements, including a broader representation of functions and an enhanced curation process. These improvements aim to increase both the quality of annotations and their coverage at the sequence level. Higher coverage has been achieved by adopting additional evidence codes. Quality of annotations has been improved by systematically applying Minimum Information About Disorder Experiments (MIADE) principles and reporting all the details of the experimental setup that could potentially influence the structural state of a protein. The DisProt database now includes new thematic datasets and has expanded the adoption of Gene Ontology terms, resulting in an extensive functional repertoire which is automatically propagated to UniProtKB. Finally, we show that DisProt’s curated annotations strongly correlate with disorder predictions inferred from AlphaFold2 pLDDT (predicted Local Distance Difference Test) confidence scores. This comparison highlights the utility of DisProt in explaining apparent uncertainty of certain well-defined predicted structures, which often correspond to folding-upon-binding fragments. Overall, DisProt serves as a comprehensive resource, combining experimental evidence of disorder information to enhance our understanding of intrinsically disordered proteins and their functional implications. © The Author(s) 2023. Published by Oxford University Press on behalf of Nucleic Acids Research.},
note = {Cited by: 10; All Open Access, Gold Open Access},
keywords = {},
pubstate = {published},
tppubtype = {article}
}
Hamidreza Ghafouri; Tamas Lazar; Alessio Del Conte; Luiggi G. Tenorio Ku; Peter Tompa; Silvio C.E. Tosatto; Alexander Miguel Monzon; Maria C. Aspromonte; Pau Bernadó; Belén Chaves-Arquero; Lucia Beatriz Chemes; Damiano Clementel; Tiago N. Cordeiro; Carlos A. Elena-Real; Michael Feig; Isabella C. Felli; Carlo Ferrari; Julie D. Forman-Kay; Tiago Gomes; Frank Gondelaud; Claudiu C. Gradinaru; Tâp Ha-Duong; Teresa Head-Gordon; Pétur O. Heidarsson; Giacomo Janson; Gunnar Jeschke; Emanuela Leonardi; Zi Hao Liu; Sonia Longhi; Xamuel L. Lund; Maria J. Macias; Pau Martin-Malpartida; Davide Mercadante; Assia Mouhand; Gabor Nagy; María Victoria Nugnes; José Manuel Pérez-Cañadillas; Giulia Pesce; Roberta Pierattelli; Damiano Piovesan; Federica Quaglia; Sylvie Ricard-Blum; Paul Robustelli; Amin Sagar; Edoardo Salladini; Lucile Sénicourt; Nathalie Sibille; João M.C. Teixeira; Thomas E. Tsangaris; Mihaly Varadi
PED in 2024: improving the community deposition of structural ensembles for intrinsically disordered proteins Journal Article
In: 2024, ISSN: 03051048, (Cited by: 10; All Open Access, Gold Open Access).
@article{Ghafouri2024D536,
title = {PED in 2024: improving the community deposition of structural ensembles for intrinsically disordered proteins},
author = { Hamidreza Ghafouri and Tamas Lazar and Alessio Del Conte and Luiggi G. Tenorio Ku and Peter Tompa and Silvio C.E. Tosatto and Alexander Miguel Monzon and Maria C. Aspromonte and Pau Bernad\'{o} and Bel\'{e}n Chaves-Arquero and Lucia Beatriz Chemes and Damiano Clementel and Tiago N. Cordeiro and Carlos A. Elena-Real and Michael Feig and Isabella C. Felli and Carlo Ferrari and Julie D. Forman-Kay and Tiago Gomes and Frank Gondelaud and Claudiu C. Gradinaru and T\^{a}p Ha-Duong and Teresa Head-Gordon and P\'{e}tur O. Heidarsson and Giacomo Janson and Gunnar Jeschke and Emanuela Leonardi and Zi Hao Liu and Sonia Longhi and Xamuel L. Lund and Maria J. Macias and Pau Martin-Malpartida and Davide Mercadante and Assia Mouhand and Gabor Nagy and Mar\'{i}a Victoria Nugnes and Jos\'{e} Manuel P\'{e}rez-Ca\~{n}adillas and Giulia Pesce and Roberta Pierattelli and Damiano Piovesan and Federica Quaglia and Sylvie Ricard-Blum and Paul Robustelli and Amin Sagar and Edoardo Salladini and Lucile S\'{e}nicourt and Nathalie Sibille and Jo\~{a}o M.C. Teixeira and Thomas E. Tsangaris and Mihaly Varadi},
url = {https://www.scopus.com/inward/record.uri?eid=2-s2.0-85181761325\&doi=10.1093%2fnar%2fgkad947\&partnerID=40\&md5=0ad51562357f3e5f603d744e02f8729a},
doi = {10.1093/nar/gkad947},
issn = {03051048},
year = {2024},
date = {2024-01-01},
abstract = {The Protein Ensemble Database (PED) (URL: https://proteinensemble.org) is the primary resource for depositing structural ensembles of intrinsically disordered proteins. This updated version of PED reflects advancements in the field, denoting a continual expansion with a total of 461 entries and 538 ensembles, including those generated without explicit experimental data through novel machine learning (ML) techniques. With this significant increment in the number of ensembles, a few yet-unprecedented new entries entered the database, including those also determined or refined by electron paramagnetic resonance or circular dichroism data. In addition, PED was enriched with several new features, including a novel deposition service, improved user interface, new database cross-referencing options and integration with the 3D-Beacons network\textemdashall representing efforts to improve the FAIRness of the database. Foreseeably, PED will keep growing in size and expanding with new types of ensembles generated by accurate and fast ML-based generative models and coarse-grained simulations. Therefore, among future efforts, priority will be given to further develop the database to be compatible with ensembles modeled at a coarse-grained level. © The Author(s) 2023. Published by Oxford University Press on behalf of Nucleic Acids Research.},
note = {Cited by: 10; All Open Access, Gold Open Access},
keywords = {},
pubstate = {published},
tppubtype = {article}
}